Integrating AI Into the Anatomy of Autonomous Networks
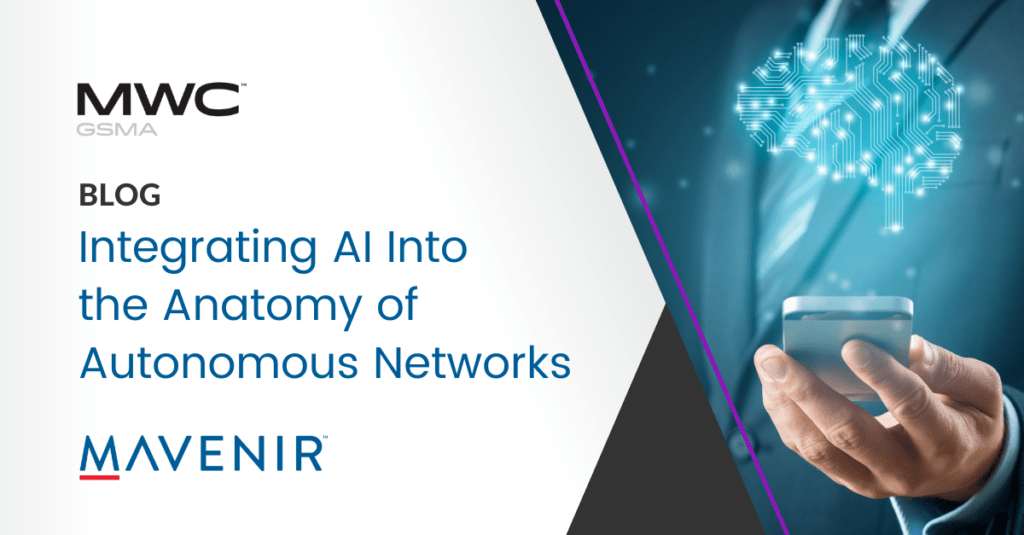
One of the key themes at this year’s Mobile World Congress (MWC) was ‘Connecting Everything.’ According to GSMA Intelligence, by 2030 there will be 6.3 billion unique mobile subscribers globally, with five billion global 5G connections. In one of the morning keynotes, CEOs were talking about the exciting future of networks – what we can connect, what we can enable, etc. This vision of connecting everything comes with its own challenges of managing the complexity of these future networks that will be delivering a different quality of service (QoS) to different end users demanding different price points, all in real time. To manage that growing complexity of meeting such a heterogeneous and dynamic service demand, future networks need to be more autonomous. In a keynote at the MWC 2024, I took part in ‘The Anatomy of Autonomous Networks’ panel, discussing the nuts and bolts of an autonomous networking – what it takes to automate a network and how to go beyond automation to make the network autonomous by introducing Machine Learning (ML), a form of Artificial Intelligence (AI). In this blog, I capture key points of my talk at this panel discussion.
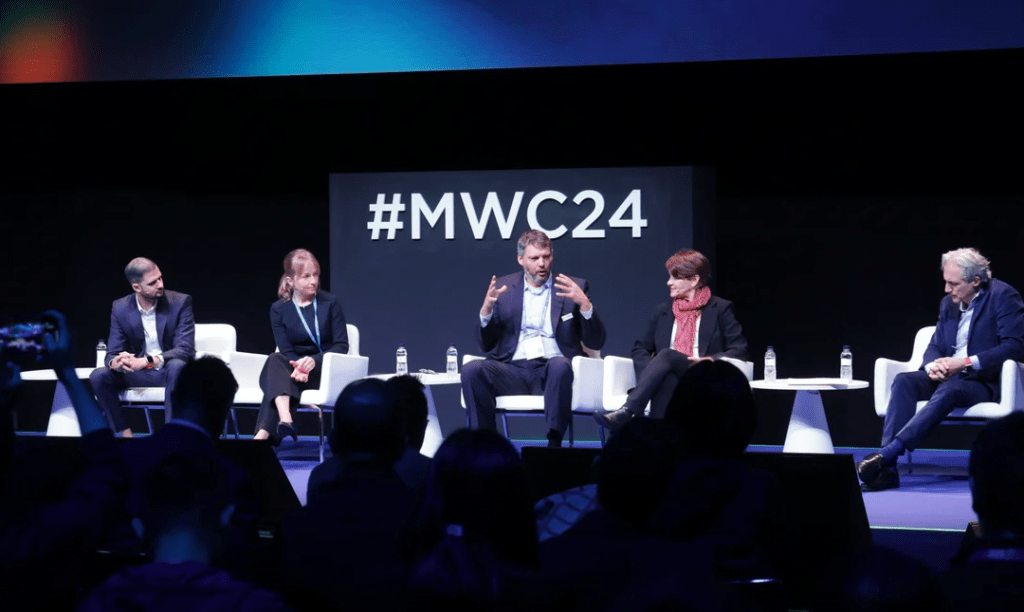
To realize autonomous networks, operators must embrace Artificial Intelligence (AI)/Machine Learning (ML) in network operations. Many operators have already started the journey to autonomous networking through automation, but AI/ML will take their network autonomy to the next level – above and beyond what an operations person or team can do. AI/ML can crunch much more data than a human brain alone and learn the patterns in that sea of data to unearth insights and determine best cause of action, given a certain state of the network. AI/ML can be used to:
- Determine causation (root cause) for observed network behavior
- Forecast what will occur in the network, so proactive action can be taken
- Take autonomous action to achieve a desired network state based on local network conditions
- Personalize service giving different QoS to different end user devices
Of course, operators will want to leverage the power of AI/ML to elevate their operational capability, but there is a challenge. The autonomous aspect means AI/ML will be making changes on the network on its own and this is perceived as operational risk. With an advanced AI technique such as Deep Q Learning, the AI/ML will be taking actions to explore or learn its environment before it can use these learning to exploit or achieve its target. To mitigate this risk, we can simply apply another AI/ML technique to the solution. We can use a form of Gen AI called Generative Adversarial Networks to create a “deep fake” of the network. This deep fake is general representation of the network based that has been learned from the network data itself. With this model, changes from the Deep Q Learning model can be tried on the deep fake before applying to live production. This is, in essence, a Digital Twin of the network that can be used for offline validation of any action an AI/ML model will take.
To build autonomous networks, operators need to do more than look at the technology aspects of AI/ML alone. They need to create organizations or teams that have a blend of skillsets spanning Cloud, Data Science and Telco domains.
- It starts with the Telecom domain experts, who understand the problem that needs to be solved and know what data is relevant to the problem
- The data scientists understand how to process the data and transform raw telco data into something that machine learning algorithms can understand. They select the ML algorithm suited for the problem, do the hyperparameter tuning, training and validation.
- The cloud experts play a key role in data collection, which is very important in AI/ML. They also build the automation framework which provides the control action capability for the AI/ML to take action.
To effectively implement AI in Telecom, onboarding people with above skillsets is a start but not the end. There is also a need for an environment that allows them to work collaboratively on AI/ML. Experts from the telecom, cloud, and data science domains use different problem-solving approaches due to their different backgrounds and simply don’t always look at things the same way. It takes time for them to understand each other and for their views to align on how to solve the problem. Creating such a work environment and culture is easier said than done. It requires more than putting everyone on the same team or in the same room. It requires the team to align on a shared vision and share some joint success, so they learn to grow together. And once they start getting to that point, that is when the magic truly starts to happen.
*Blog by Brandon Larson, Senior Vice President and General Manager of Mavenir’s Cloud, AI & IMS Business Strategy