How Standards-Based AI-Powered Analytics Drive Network Automation and Business for CSPs
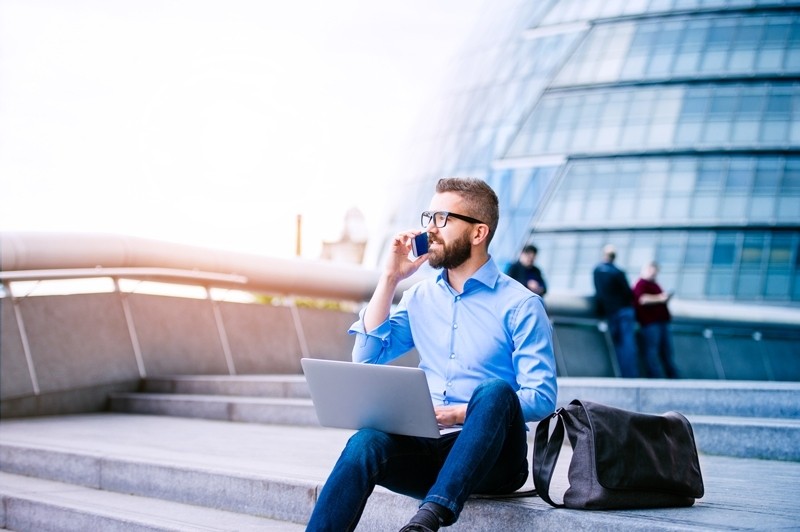
AI-Powered Analytics – A Key Business Enabler
The increase in network costs and near flat revenue from connectivity services causes Communications Service Providers (CSPs) to explore opportunities beyond traditional services. As CSPs embark on a journey to transform themselves into Digital Service Providers (DSPs), a key enabler for success will be network automation powered by Analytics and Artificial Intelligence/Machine Learning (AI/ML) as part of 5G deployments.
Various trials and early non-standard deployments of AI and analytics powered network automation and operations have proven to reduce capex by ensuring efficient network resource utilization. CSPs can also achieve opex reduction in network operations through analytics powered closed-loop automation.
Additionally, Analytics powered network automation enables the quick rollout of Service Level Agreement (SLA) bound differentiated services and granular control to tap new opportunities such as security as a service and zero-touch private mobile networks with industry vertical automation support using Edge AI.
Despite these business benefits, AI and Analytics powered network automation was viewed as a complex and difficult milestone to achieve in a CSP network due to missing standards. Industry standard bodies, including 3GPP and the O-RAN Alliance, have addressed this concern by defining specifications and standard analytics functions to enhance network procedures and provide more control on Radio Access Network (RAN) management. Specific standards-defined analytics functions for Network Automation include Network Data Analytics Function (NWDAF) and the RAN Intelligent Controller (RIC).
How AI and Analytics Powered Network Automation Works
The way AI-powered Standard Analytics functions such as NWDAF and RIC integrate and interact with the network ecosystem can be classified into three different modes of operations. These modes are designed to meet the various scale and end-to-end automation needs in the network. To simplify the complex, let’s examine each mode and compare it to services offered by a stockbroker for easier understanding.
Mode 1: Analytics as a Service
NWDAF works in such a way that it provides other Network Functions (NFs), including Application function and Operations Administration and Maintenance (OAM), that can be a data source and analytics consumers with requested Analytics services. It does not instruct, recommend, or demand an action out of the analytics provided to them, so the analytics consumer can compare multiple analytics outputs provided, such as UE Mobility and UE communication analytics to make a network configuration or policy change.
This “light mode” of working in NWDAF ensures that it can handle volume, velocity, and variety of analytics services requested by 5G NFs are met. Also, in the NWDAF case making a recommendation or action as output would make it “heavy” as it needs to have different logics and stacks from different NFs.
This mode of the analytics function operation can be compared to a stockbroker’s analysis report for short, medium, and long-term projections based on the stock name. The buy/sell/hold decision for the stock rests with the customer or researcher, who can also access multiple analysis reports from different stockbrokers to decide and execute the final action.
Mode 2: Analytics-Based Action Recommendation
RIC performs the radio parameter optimizations and Radio Resource Management (RRM) through RAN functions Central Unit – Control plane (CU-CP), Central Unit – User Plane (CU-UP), and Distributed Unit (DU), which are often referred to as E2 nodes. Based on historical data and ML model predictions with current data, a RAN control decision is sent to E2 nodes. However, the decision of whether to execute or discard (which is rare) lies with the E2 node as they also execute conflict management to handle multiple related change requests.
Non-real-time RIC mostly operates in this mode and as it is a RAN-specific analytics function making inbuilt logics to provide recommended actions as output makes sense as this helps keep other RAN functions simpler. This also gives backward compatibility to upgrade SON implementations. Recommendation instead of control is preferable in cases where E2 nodes need to consider multiple factors along with RIC recommendation to decide on an action.
This mode of operation can be compared to a stockbroker’s trading recommendation service in which a broker can recommend the customer to buy, sell, or hold a stock, but the decision to execute the recommendation still ultimately lies with the customer.
Mode 3: Analytics-Based Control Action
Near-real-time RIC is currently enhanced in standards to take over the execution of RRM control loop instead of an E2 node based on granular Analytics decision at UE level. Control decision in Near-RT RIC is based on analytics prediction using current E2 data received at UE/service level granularity along with policy, intent, and ML model trained using historical data received from Non-real-time RIC.
RAN control actions instead of recommendations help in low latency RAN control and enable critical use cases, such as SLA assurance, mMIMO beamforming optimization, real-time mobility, and robustness optimization.
This mode of operation can be compared to a stockbroker’s wealth management service in which the broker manages the customer’s fund and executes the trade on behalf of their customers. Here, the customer provides needed authorization and control to make stock trading decisions in their account.
It is Time for the Transformation Journey
While mode 1 ensures smart decisions by other 5GC NFs using NWDAF services, modes 2 and 3 supported by RIC enables near real-time granular RAN control making closed-loop, zero-touch network automation a reality. An ROI-driven, standards compliant, phased approach to the implementation of all three modes by use case will help CSPs successfully launch new services and achieve efficiency and savings.
As NWDAF and RIC become more standard and promising with industry collaboration addressing gray areas in newer releases, it is time for CSPs to embrace these analytics functions to reduce costs, drive ROI, and enable new services with intelligent dynamic orchestration to truly transform into DSPs.
Learn more about Mavenir AI and Analytics solutions that will help drive the transformation journey.